Creating Data Masterpieces: Our Investment in Deepnote
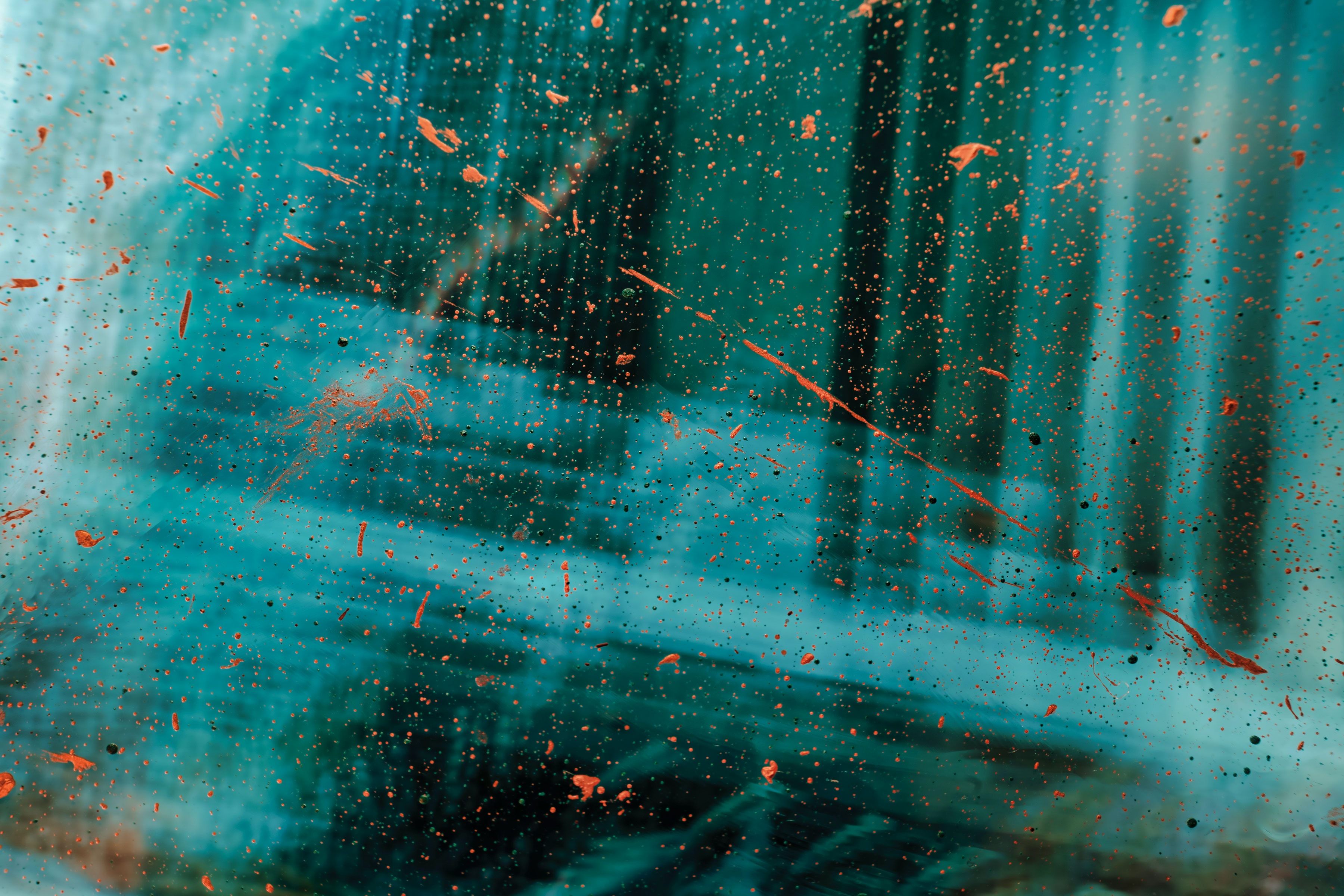
It is no secret that data is going through a renaissance. Once the closely held competitive advantage of some of the world's leading businesses, the production and consumption of data have become a part of our everyday lives. From the steps we track on our wrists, to the ads we see on our smartphones, data is at the center of not just the modern economy, but the modern life. It’s pretty hard to imagine living without it.
"We have a lot of new paint and a rapidly increasing number of artists, but we are still using paintbrushes and techniques from the 18th century"
— Bryan Offutt, Index Ventures
And the world has had to change to keep up. Demand for skilled labor in data-related roles has skyrocketed over the past decade, as companies look to keep up with the demands of competition in a data-oriented economy. Universities around the world have responded accordingly, pivoting large portions of their curriculums (and budgets) to focus on data literacy. Meanwhile, organizations like Confluent, Snowflake, and MongoDB (not to mention AWS, Azure, and GCP) have built multi-billion dollar businesses selling the infrastructure that constitutes the foundation of the data world.
But data collection and skilled labor are only part of the battle. In order to be useful, data needs to be analyzed. Otherwise, it just sits there. We have a lot of new paint and a rapidly increasing number of artists, but we are still using paintbrushes and techniques from the 18th century.
From Snapshot to Narrative
Thankfully, a number of tools have come to help solve these problems in recent years, few of which are more popular than the Jupyter notebook. The underlying principle of the Jupyter notebook is both simple and brilliant: that effective data analysis is not just about presenting numbers, it’s about telling a narrative. And stories can’t be told with just numbers and graphs. They need context. By allowing data practitioners to mix code, the output of that code, written text, graphs, and even error handling into a single document like user experience, Jupyter hoped to take data analysis from a single, murky snapshot to a rich motion picture.
And the market seems to have agreed with the approach. Originally spun out of the iPython project in 2014, Jupyter has seen enormous adoption over the past 15 years. Just take a look at the latest estimate of Jupyter notebooks on Github here. Spoiler: it’s grown nearly 10x since 2017, with nearly 2,500 being added per day. Clearly, Jupyter was on to something.
From Narrative to Blockbuster
Yet, for all of its positive qualities, Jupyter is not without its faults. It can be clunky and awkward as a user experience. Keeping track of state is difficult and error-prone. And, most notably, it’s largely single-player. Using Jupyter notebook is a bit like being stuck in the .docx age, if sharing a word document also required your colleague to have their computer’s environment set up exactly correct before they could open anything. Jupyter did a decent enough job giving a new crop of artists the brushes they need to make beautiful data paintings. But data analysis, like paintings, needed something else: an audience. And this is where Jupyter notebooks fall very, very short.
I started thinking about this a lot in the early months of 2019. It was becoming very clear that the importance of data within companies was growing exponentially, and so too was the importance of the data practitioners responsible for it. In my mind, this change had very clear parallels to what had started to happen with design just a few years earlier. Like designers, data teams were no longer magical wizards building models (or mock-ups) off on their own, they were business-critical centerpieces of the org. Yet, unlike designers, data practitioners they were still stuck working in single player Jupyter, or “data’s Adobe Illustrator”. It was clear that what data practitioners needed was their own “data Figma”: a modern, collaborative, cloud-based tool that allowed them to efficiently share their work not just with one another, but with the entire organization.
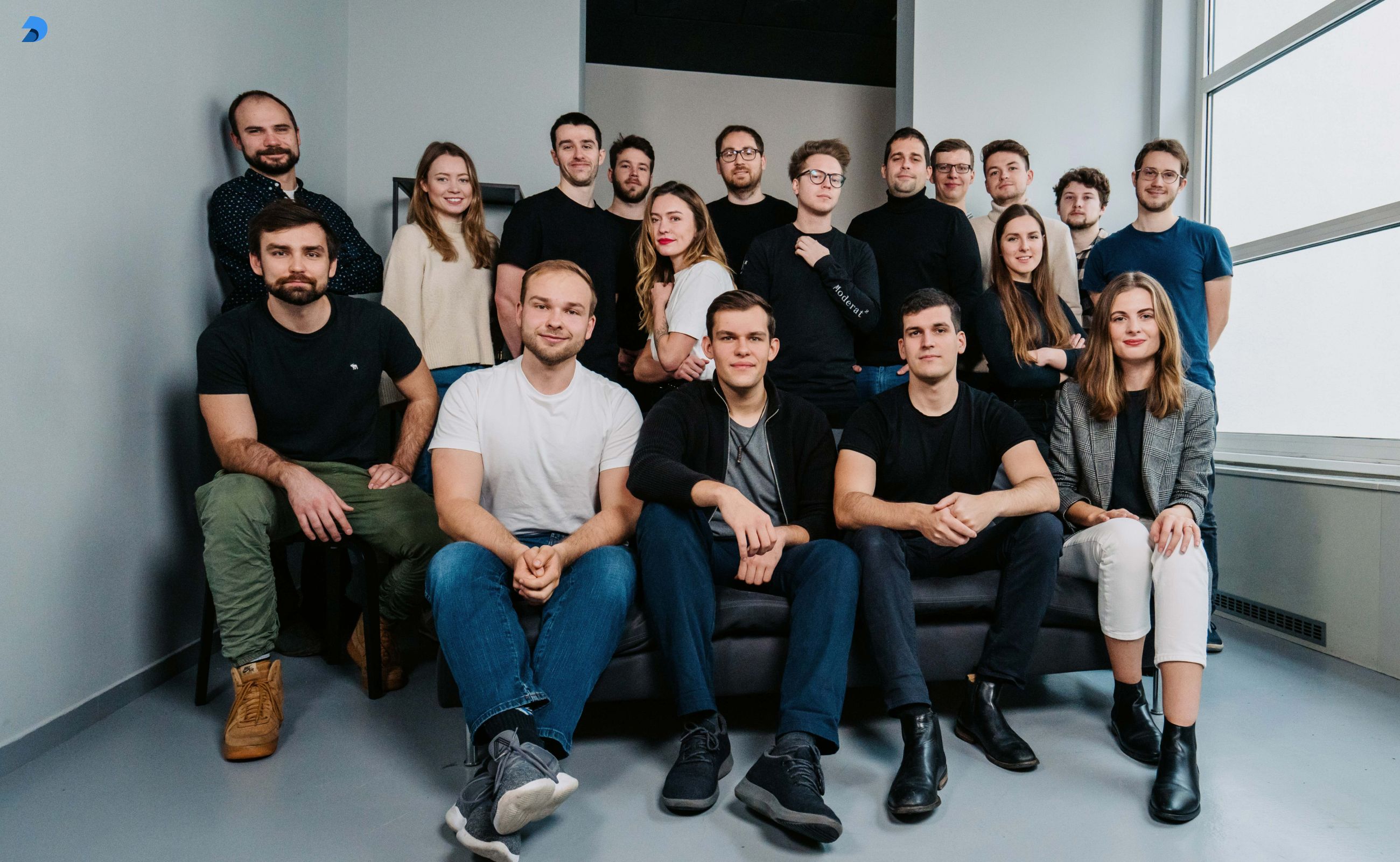
The Deepnote team
From Prague With Love
Luckily, I did not have to wait long. Just a few short months later in August of 2019, I heard from my colleague Nina about this hot YC company called “Deepnote”. I took one look at the product and knew I had found what I was looking for. I was blown away by what the team had managed to build in just a few short months. The attention to detail and simplicity in the product experience was superb, and they had already solved so many of the biggest pain points of Jupyter: live collaboration, easy to spin up cloud compute, commenting, a variable explorer, and saved environments so that your colleagues or friends could start exploring your work with zero set up on their end.
"Like data analysis, building the future of data is not a solo endeavor, but a team sport. And we are delighted to be on team Deepnote."
— Bryan Offutt, Index Ventures
As seems fitting for a product-led company, I met the Deepnote team shortly after falling in love with the product. I came in with high expectations and was delighted to find that they were met and surpassed. What I had seen in the product was just the beginning of Jakub and the team's vision for Deepnote. Jakub walked me through his real vision: to empower data practitioners everywhere to easily share their work not just with one another, but with the world. About 72 hours following that first product experience, we were delighted to have the ink dry on co-leading Deepnote’s Seed round with our good friends at Accel.
And the progress since then has been astounding. The company has grown from a few folks in Prague to a team of nearly 30 strong, and added amazing new features to the product like Notion embeddings, easily shareable dashboards, and a totally revamped experience for teams. But, this is just the beginning. Like data analysis, building the future of data is not a solo endeavor, but a team sport. And we are delighted to be on team Deepnote.
Published — Jan. 31, 2022