How Adaptive is Bringing GenAI Alignment to the Enterprise
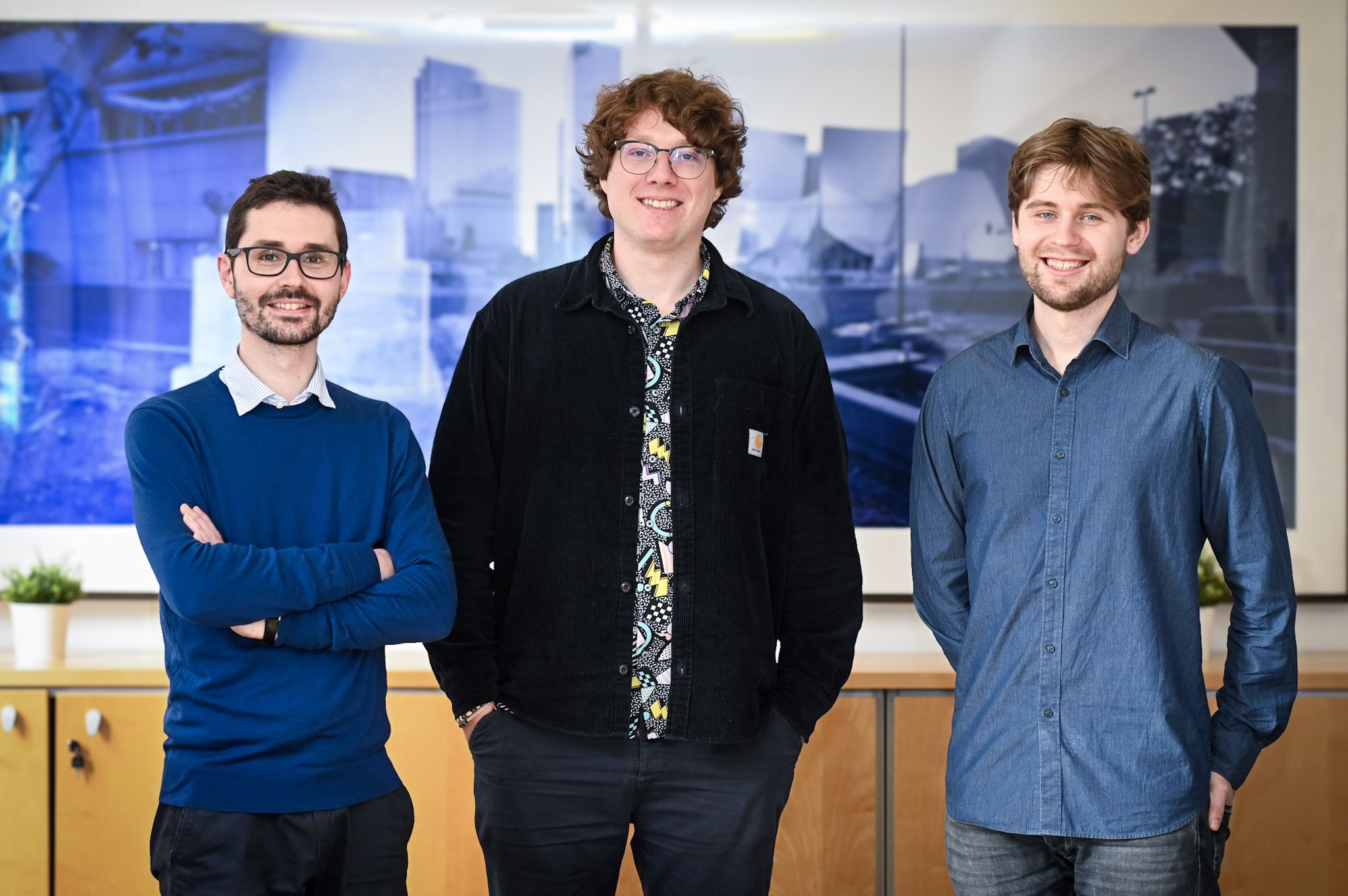
The Adaptive ML founding team: Baptiste Pannier, Julien Launay, and Daniel Hesslow.
The consumer internet as we know it today is built around personalization.
Over the past decade, companies like TikTok, Netflix, and Twitter have mastered the art of delivering what can seem like an endless stream of videos and text. But why do we get sucked in? And what makes it feel like we each have our own “copy” of the internet? The answer is simple: recommender systems. These systems unlock the power of the internet for individual consumers, allowing companies to provide seamless, personalized experiences that replace the generic, unfeeling nature of the “old” internet with a new feeling of warmth and familiarity.
Generative AI, it turns out, isn’t all that different. Just as consumer internet companies have enabled scalable human content generation, GenAI is giving rise to machine-generated content. We query these models for information on new restaurants, advice on how to write emails, images of puppies riding rockets, and more. However, these machine-generated responses often lack the warmth and humanity we’re used to finding on the consumer internet. So how do we imbue “warmth” into GenAI products? And more importantly, how do we make engaging with a GenAI model feel like a singular, personalized experience?
Industry labs like OpenAI and DeepMind have attempted to solve this problem using alignment-based techniques, also known as preference tuning. These techniques incorporate human feedback and guide models to generate more customized and “warm” responses. Preference tuning was pioneered by multiple labs, including OpenAI, and was used to uplevel raw GPT models, leading to the creation of ChatGPT. But while preference tuning is extremely powerful and necessary to create production-grade experiences, it requires deep expertise to properly implement. Most enterprises lack the necessary talent and infrastructure to tune their models, leaving them stuck deploying limited GenAI experiences.
This is exactly the problem Adaptive is trying to solve. Adaptive is productizing alignment techniques to allow every enterprise to deploy models that deliver personalized experiences to customers. Today, most enterprises rely on base models to power their GenAI experiences. However, there is a lack of tooling to help enterprises upgrade these models from their out-of-the-box functionality to provide true production-grade experiences. The core challenge lies not just in having the models return results that are technically correct, but in making those results feel personalized and human.
This is where alignment techniques come in. Different forms of preference tuning, such as reinforcement learning from human feedback (RLHF) and direct preference optimization (DPO), take into account user preferences and allow the models to understand and “align” to them. For example, in order to perform RLHF, an enterprise would need to do the following:
- Perform standard fine-tuning on the model
- Collect large volumes of preference data → have the model generate multiple responses to a given query, and have a human pick the best response
- Use this preference data to train a reward model
- Use the reward model to optimize the model
- Host the tuned model
When we first learned about preference tuning, our concerns were primarily centered around the second bullet—the question of data. How does one scale up a collection of relevant preference data? Would enterprises even be bothered with this? As we spoke with more companies, we realized enterprises were deploying GenAI models in “copilot” scenarios, where a human makes the final decision about whether or not to accept a suggestion. This is preference data—exactly what this type of tuning needs. Adaptive’s platform provides an SDK that lets enterprises easily collect these interactions and augment them using RLAIF (an alternative to RLHF that allows models to provide feedback in addition to humans).
Each of the above steps is quite complex and requires not only expertise but resources to implement. With preference tuning techniques typically restricted to industry labs, how does an enterprise go about properly training a reward model, optimizing the end model, and hosting it? Adaptive combines these steps in a single platform that updates the model on a regular basis, incorporates feedback, and provides visibility into the preference-tuning process so that enterprises maintain control over their product and can directly optimize for their business objectives. Ultimately, Adaptive’s enterprise-grade product allows companies to ship tailored GenAI experiences, bringing the power of recommender systems to GenAI.
The complexity of Adaptive’s platform requires a team with a deep understanding of what it takes to build GenAI models (on both the engineering and scientific sides), systems, and enterprises. The Adaptive team led the development of the open-source Falcon LLM models, deployed LLMs with enterprise customers at LightOn, and has been working together in scrappy environments for many years. Their depth of experience across multiple dimensions has given the company a strong foundation to serve enterprise needs at scale. We’re excited to partner with the Adaptive team as they help enterprises harness the power of alignment techniques and deliver production-grade GenAI experiences to customers.
Published — March 11, 2024