Index OnAir: The Future of Robotics
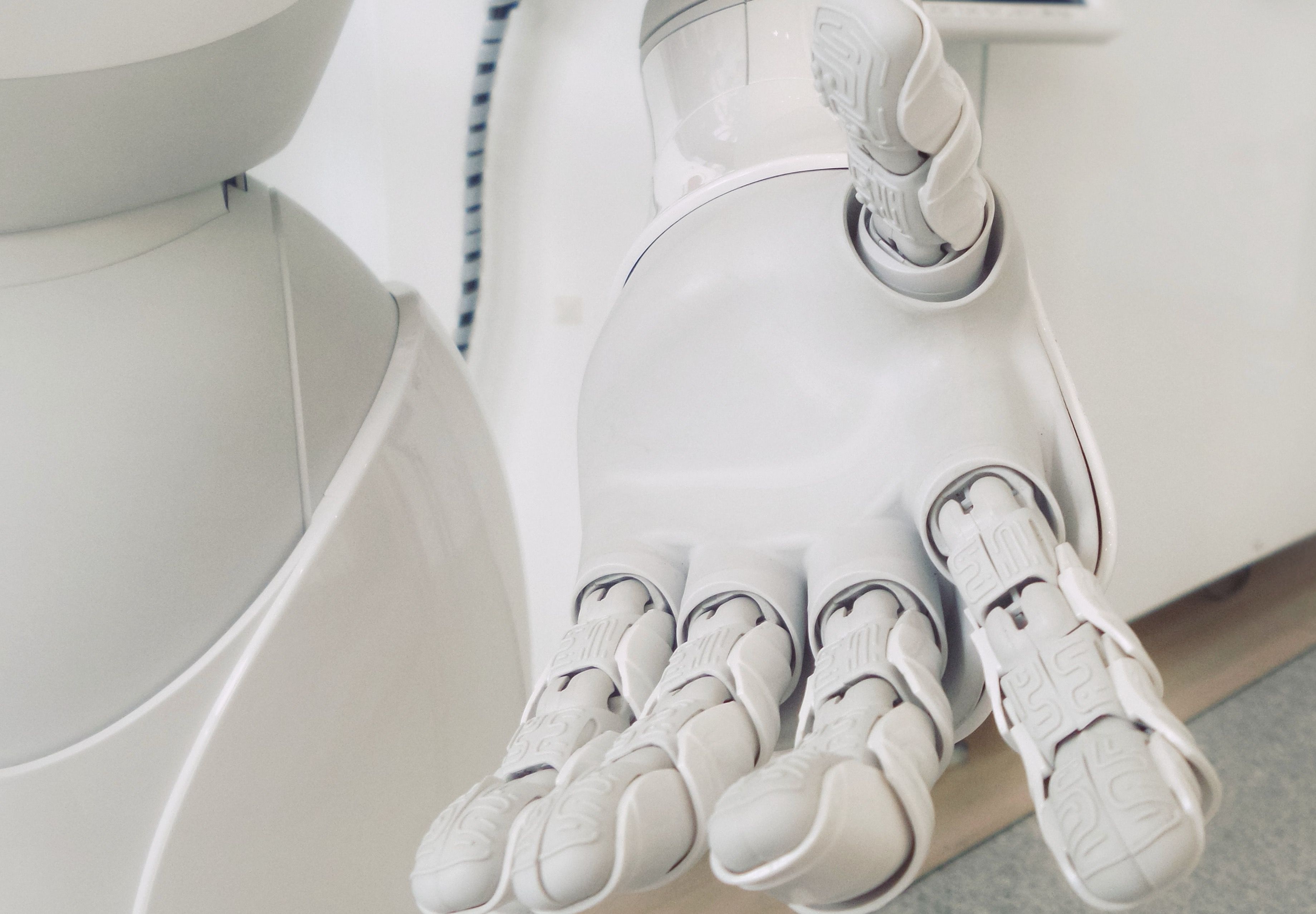
Both Aurora and Covariant are on the cutting edge of machine learning that has a powerful real-world impact. Aurora, founded in 2017 and led by Chris Urmson, is focused on delivering the benefits of self-driving technology safely, quickly, and broadly with the Aurora Driver. Covariant, Co-Founder and CEO Peter Chen explains, is creating a universal AI for industrial robots that enables them to learn and adapt to different environments and across different industries. Wired’s senior writer Will Knight moderates the discussion.
Will knight: Why have we seen such a fundamental shift to automation over the last five to ten years?
Chris Urmson: The idea of automation in automobiles is not new. Incremental developments have included not having to hand crank your starter, and automatic shifting. Even the idea of a vehicle driving itself is getting over 100 years old; there was a sketch in Scientific American from 1918 that showed a driverless car.
There are several reasons autonomy is finally becoming viable, including the advancement of sensor technology. Cameras have been driven by the rapid pace of innovation in the cellphone industry, and we’ve seen exciting breakthroughs in LiDAR, including at Aurora. We’ve had advancements in computing, achievements in machine learning, and the amount of computation that is available to extract meaning from massive amounts of data. Vehicles themselves have become more amenable to automation, with a higher density of electronics and being easier to control than even a decade ago.
We’ve also seen an increased understanding that the status quo is just not acceptable. We lose 40,000 Americans every year on our roads, and 1.2 million people globally — it’s a major blight on society.
peter chen: We unapologetically believe in the power of machine learning. There have been amazing strides in the development of the sensors, but the underlying technologies have existed for decades. It’s modern-day machine learning that is enabling the layer of intelligence that powers what we’re doing now. We saw that with AlphaGo — those leaps forward have been led by developments in machine learning. Machines can make decisions better than humans.
It’s modern-day machine learning that is enabling the layer of intelligence that powers what we’re doing now.
And the ecommerce boom has created large demand on the logistics backbone. When you have a distribution format where goods are routed to tens of thousands of retail stores and people go to stores to buy it, that’s very different to shipping to tens of millions of people. It’s an order of magnitude more complex, and that demand has driven innovation and labor scarcity. When people talk about automation they think of robots taking people's jobs, but in the very near term every customer we talk to in warehousing and logistics say actually there aren’t enough people who want to do those jobs.
Will: Putting robots out in the real world to deal with humans is clearly challenging to do, so what’s your technical approach?
Peter: The crucial question is how well can you handle the long-tail edge cases. No one wants a robot or a car that works for 10 mins and then needs someone to fix it. The level of autonomy is fundamental to this question of how you handle all those scenarios. The framework we use is to characterise what can and can’t happen, and try to simulate as much as possible of what can happen.
Simulation is powerful because it gives us a way to deal with combinatorial complexities. We’d do a first-principles analysis of what could happen, and then use the simulation to explore that space. It’s not really about making a better AI — it’s about getting better data. If it has seen more problems, it can be smarter.
It’s not really about making a better AI — it’s about getting better data. If it has seen more problems, it can be smarter.Peter Chen,
Co-Founder and CEO, Covariant.ai
Chris: We process hazard and accident analysis so that we can see where there could be failures, and then failure mode analysis to see what can break and how to mitigate that. Then we use offline simulation tools where we can create interesting events and numerous variations around those events.
You may not see a child running into the road in a lifetime of driving, but it’s still an event that matters. So we create a simulated child and generate sensor data around that — different children, different clothes, different speeds, different places — so that we start to build confidence that we would be able to react to that child in the real world. Then we’d move to structured outdoor testing and more collection of evidence.
Will: How closely are you tracking what’s going on in the machine learning world?
Peter: The first main challenge is generalization — when you put an AI into a new scenario how does it deal with it? The second is capacity: if I put you in this environment and have given you data, can you learn to master it? We want to make better models and better algorithms so we get better behaviour the first time. And with higher learning captivity, so they can take in data and learn from it.
In both these areas research is instrumental. It’s a core part of Covariant’s thesis, and why we have amazing research science working on these problems. We are tracking the academic field closely, but also working on our own theories.
Chris: One of the great cultural things about deep learning is that even work in the corporate world has been published. That came from the way deep learning moved into the industry; it was clear that for a lot of people doing important work in academia, the only way companies could entice them was to give them the flexibility to publish their work. As a community, we have all benefited from that.
Will: Is your approach to go after niche applications, or more generalized markets?
Chris: Our approach is to find the minimum [viable product] that we can make to deliver value in the world, and then expand out from that. Transportation is a multi-trillion dollar industry, and a lot of that is moving people and goods on the ground. We want to start with apps that demonstrate safety, quality, and return. We’ve invested heavily in trucking as our first industry. The cargo doesn’t get frustrated if you take a little longer to get there on a safer route, so it’s an interesting way to marry deployment with customer demand.
Peter: We’re creating a platform, but we’re also starting with a very specific application that’s ready to go and has a straightforward expansion path in an industry experiencing a lot of pain points.
When we think about building AI for the physical world, we have the interesting property of generality in that there's only one physical, three-dimensional world. If I wanted to build an IOT AI company that interprets the data stream from a fridge to an HVAC system, there’s no common ground between those systems. But trucking and taxis both need to see the world in 3D to predict where the products are going, and thinking about the affordances of interacting with objects. Once you conquer the first use case, it’s not a linear effort to the second one — it’s probably only 20% additional effort because of the strong transfer of the AI grounded in the physical world.
Will: When can we expect full autonomy?
Peter: If you asked when a robot could manipulate something as well as a human, that might be a decade away. But if you asked when we could have a robot that works as well as a human on a subset of things in a more restricted scenario, I’d say we achieved that yesterday. Some people in Europe and North America are already getting packages that were handled by Covariant robots.
It comes back to the platform concept. We can’t solve everything at once, so we find more restricted problems to get us started, and then improve dexterity, the level of manipulation, and the generality of the system over time.
Chris: If you live in a suburb of Phoenix, you can call a car that will pick you up, without a driver, and take you wherever you want to go in that neighborhood. That’s a subset of self-driving cars that's already happening. If you arrive at London Heathrow airport you can take a shuttle with no driver. Over the next decade, we’ll see a ramp of applications and vehicles operating in more diverse environments.
On the surface, we’re building a driver that will do what drivers do in the real world. But in the real world drivers do a bunch more things, like checking the load or maintaining inventory. So figuring out exactly how we interface with our partners to support their businesses will take a while. As a company we have to spend the next few years building the core product so we can build the real business on top of it.
Will: 5G promises high speeds, low latency and high reliability, but how important is it to take computation off the device?
Peter: Cloud computing is the idea you have access to a massive amount of computing infrastructure, and enables machine learning from the perspective of training and building the AI models. The combination of the cloud and 5G means that we can choose how much of that inference happens locally and how much on the cloud. We still see a lot happen locally because decisions have to be taken super quickly.
Another point is how different robots connect and communicate with each other. Even in our space the robots are connected — they share the same brain, because the training happens in the cloud and gets downloaded to each robot.
Chris: 5G won’t be truly ubiquitous. If we’re driving and hit a coverage black spot, the vehicle must be able to operate safely. So we expect inference and core-driving capability to be onboard.
However, the ability to call for help could accommodate more latency, whether that’s a person on the other end or a diagnostic system. And there’s an interesting opportunity with vehicle-to-vehicle communication too where you get similar characteristics as 5G, and could overlay that information. It would bring us to a challenge of trust — do I believe that data, or is from that an adversary? So we have to work through that complexity.
Will: How will robotics and general AI affect workers?
Chris: I absolutely believe in the long-term social benefits of making the roads safer, cheaper, and increasing accessibility. In the same way the industrial revolution improved the quality of life on the planet, autonomous driving will improve our quality of life.
I absolutely believe in the long-term social benefits of making the roads safer, cheaper, and increasing accessibility. In the same way the industrial revolution improved the quality of life on the planet, autonomous driving will improve our quality of life.Chris Urmson,
Co-Founder and CEO, Aurora
Yet while driving will be better and safer, it will be less expensive, so there will be a period of time where people who do driving jobs will retire or be replaced. It’s on us as a society to support those people through transition. A number of studies have confirmed that when you improve the efficiency of the economy, unemployment increases. But these are difficult jobs that lower life expectancy and have health issues, so it’s hard for me to see that this is not the right thing to do.
Peter: In the near term we know the problem is that there is more demand than supply. In the medium term there will be displacement, and it will be hard for a subset of people. We would like to be an active voice in policy, and in how we create change with broad support for these people. I don’t want to sugarcoat the issue of labor displacement. We need to acknowledge that impact and plan that transition. In the long-term we always benefit from productivity being unlocked, especially from repetitive work.
Will: What’s your craziest projection for the next ten years?
Chris: Automated vehicle technology is in the valley of disillusionment, and that's going to mean a massive number of companies in the space will fail. After that, we’ll start to see real success. We’re five to ten years off where we hoped we would be, but we will soon start to see the impact of self-driving vehicles and use them in daily life.
It’s an incredible time to do anything with the automotive industry because of electrification and automation — the first shift in 100 years that is fundamentally changing what it means to be in a car. The same way smartphones grew vast new businesses, this change in the cost and availability of transportation will usher in whole new businesses.
Peter: In ten years, I can say with 99.9% certainty, we will see autonomy 1.0, where any laborious tasks will be powered by AI. Then we’ll have autonomy 2.0, so the production of physical goods becomes a lot less costly and with an efficient platform built on top of it. We’re already seeing early signs of it. The analogy would be mobile 1.0, where a smartphone allows you to do anything you can do on a PC. With mobile 2.0, you have the same infrastructure but all these amazing services are overlaid on top of that platform.
Will: Do you worry that your business models are more difficult because they involve hardware?
Chris: I lament on bad days that we’re not just building a web app. The physical supply chain, the fact there’s a rate on how fast you can turn stuff out. You make high-stakes bets on technology because you work with it so far in advance, stick with it longer, and if you get disrupted it has more impact.
Peter: AI software involves so much complexity that there’s a higher barrier to entry. That gives you a more lasting advantage.
Will: What’s your biggest risk?
Peter: On the technical side, the robot is only as good as what it can do for you. We’re creating a new product where the level of autonomy, performance and ability will be critical, and we’re also defining a new market. People are not used to robots that learn and improve. Before I founded Covariant I knew there was a lot of tech innovation that would need to happen, but I didn’t know we’d also need a lot of go-to-market innovation to make that happen at scale.
Chris: This is a fundamentally hard technical problem, but the value proposition if we deliver that technology is clear. Every time we give people the chance to ride in a self-driving car they get it and they love it. So it’s getting there, and we’re delivering on it, while making sure we do it safely.
Get more insights into the start-up landscape, more Founder interviews, and Company perspectives by subscribing to our channel.
Published — Nov. 20, 2020