Un-biasing AI Machines
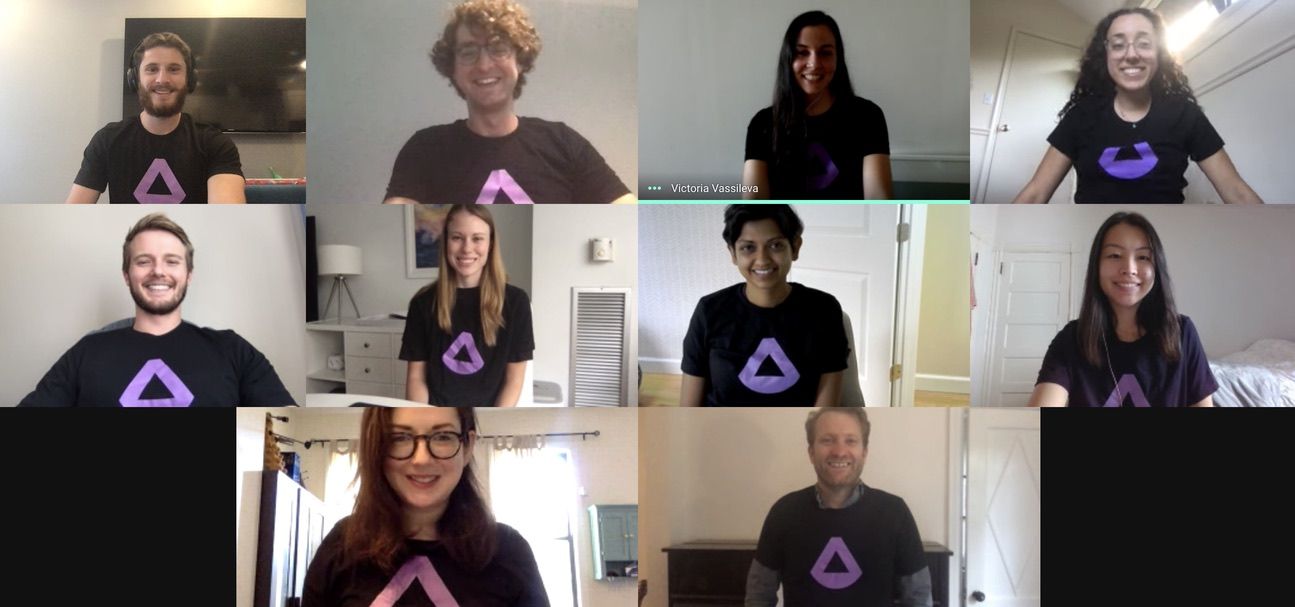
At this point, even people outside the tech industry would have likely heard a fair bit about the impact that AI technology is having on a myriad of aspects of our economy and our society. Yet, the tools available to the developers of AI-powered applications are still in their nascent stages. The early adopters of AI and machine learning technology are the tech giants: Google, Facebook, Amazon, Apple. These companies were sophisticated enough to take academic research work and build their own infrastructure to implement and productionize this new technology. Most other companies don’t have the means or the staff to assemble the requisite infrastructure to leverage AI to help their businesses. Yet, the power of the technology and the benefits it yields can provide a significant competitive edge today and will likely become table stakes tomorrow.
This is particularly problematic because AI has elements that are distinctly different from classic software development. In traditional software, the core ingredient to its effectiveness is the logic that the software embeds. After all, software is a set of commands that the developer issues to a computer. The developers inject logic into that software. AI on the other hand fundamentally recognizes patterns in data and makes predictions based on those patterns. While classical software is quite explicit in what it does and how it does it, AI appears more “intuitive” - and, in some ways, strangely human.
While the “intuition” of AI is based on mathematics (admittedly mathematics that I have long since forgotten, if I ever did understand it), AI models often appear like a “black box” to their observers. AI systems are fed data, train themselves on that data, predict what might happen, and act based on those predictions. How they predict and what affects those predictions is not at all easy to decipher. This characteristic of AI becomes challenging when AI makes mistakes.
"One of the most critical aspects of the AI stack is in the area of performance monitoring and risk mitigation. Simply put, is the AI system behaving like it’s supposed to?"
— Mike Volpi, Index Ventures
AI errors happen all the time and can be caused by a lot of issues. Sometimes the data that a system has been trained on is insufficient or faulty. Sometimes the models have “overfitted” or attempted to mimic their training data too closely. Sometimes the models have valued certain categories of the data more than they should. The particularly tricky part of AI models is that they experience something called “drift”. This is essentially a model that begins to make increasingly erroneous predictions over time. For example, when predicting the price of a house, an AI model might put too much credence on the square footage and not on the zip code. Some of these mistakes are pretty benign, but some can have extremely dangerous implications. An AI system might deny a perfectly creditworthy person a credit card. It might lead a doctor’s diagnosis of cancer astray. Perhaps, it might sentence a felon to prison for too many years. Or it could lead to substantial financial loss.
Many new companies got started to address this challenge and better harness the power of AI. While some of the earlier generations of AI infrastructure companies attempted to provide the “full-stack” of AI tools to their customers, the most recent crop tends to be more focused on specific elements of the AI stack. These companies are founded on the concept that the customers will assemble the AI stack from a series of “best-of-breed” lego blocks that, combined, represent the AI architecture of any given company.
One of the most critical aspects of the AI stack is in the area of performance monitoring and risk mitigation. Simply put, is the AI system behaving like it’s supposed to? The core aspect of this lego block is to observe a functioning AI model to check if it’s operating within reasonable boundaries and to detect how and why a model may no longer be acting “normally.” One of the key outputs of the lego block is to ensure that the model does not produce biased results - and if it does, to explain why and avoid problems like drift and bias.
At Index, we love investing in founders that are what we like to call “practitioner-entrepreneurs”. These are founders that have taken on a singular and hard-to-solve problem in their professional lives. They often develop technology to address it in a former company. At one point, they realize that many other professionals are faced with that same challenge and they build a product company to address it. Jay Kreps was a practitioner-entrepreneur when he started Confluent after developing Kafka at LinkedIn. Spencer Kimball and Peter Mattis were practitioner-entrepreneurs when they conceived of CockroachDB while still at Square. They and many more populate Index’s portfolio.
Adam Wenchel is that archetype. Adam sold his first start-up (which used machine learning for defensive cybersecurity) to Capital One where he was given the role of managing their AI center of excellence. Capital One is an early adopter of many technologies. They were one of the first financial institutions to embrace the cloud, open-source software, and yes, AI/machine learning. One of the many applications that Capital One used machine learning for was to determine the creditworthiness of their customers. Adam and his team were faced with complex regulatory and compliance requirements for this task and built a system to monitor Capital One’s machine learning systems for bias and other failure modes.
"At Index, we love investing in founders that are what we like to call 'practitioner-entrepreneurs.' These are founders that have taken on a singular and hard-to-solve problem in their professional lives."
— Mike Volpi, Index Ventures
Having seen the value of the solution, Adam decided to give entrepreneurship another go. He left a comfortable job to start Arthur.ai. I had the fortune of meeting Adam through some academic connections at the University of Maryland (he’s head of the Computer Science Advisory Board there) and learned that he had started the company along with John Dickerson, who is one of the most highly respected young professors of AI at UMD.
When Adam originally pitched me the idea for Arthur, I had to go down a bit of a rabbit hole on AI technology. Once I realized that explainability, bias, and performance monitoring were sine qua non building blocks of the modern AI stack, we enthusiastically invested in his seed round two years ago.
Since then, Adam has “brought the band back together” with a number of critical hires that he worked with at Capital One such as Keegan Hines as his VP of ML. The team also includes John Dickerson as Chief Scientist and Liz O’Sullivan as VP of Commercial. The team has shipped a product and captured several impressive customers - especially for a company in the seed stage.
Today, we are proud to announce that Index Ventures will be leading the Series A round for Arthur. Adam and his team have impressed us immensely during the last year and a half and we couldn’t be more excited to sign-up for the rest of their journey. We have enormous confidence that Arthur will become an integral component of the modern AI stack and that they will empower many companies and organizations to better harness the power of AI.
Published — Dec. 9, 2020