Understanding the Potential and Limitations of AI
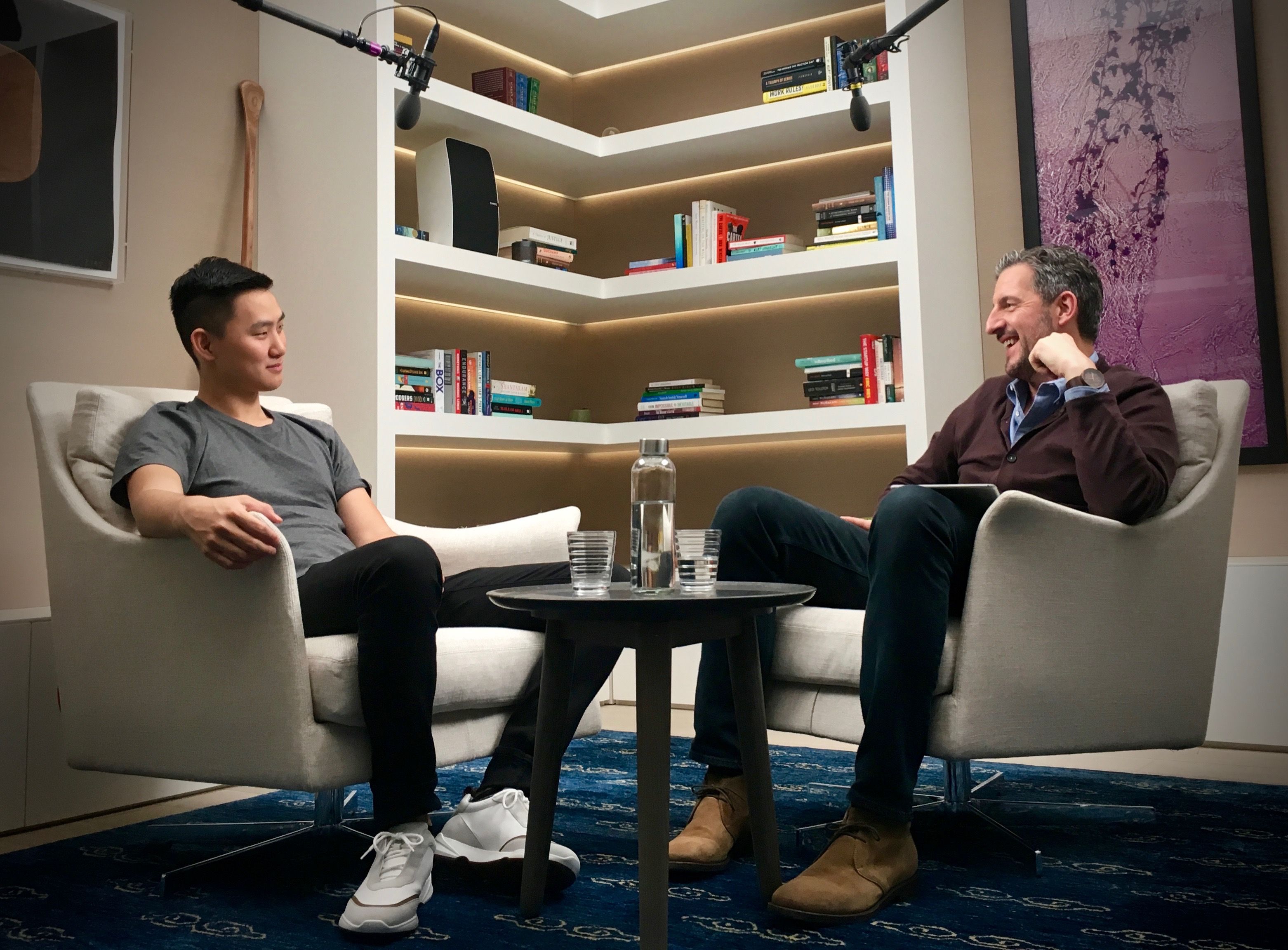
Alex Wang founded Scale in 2016, seeing the vast potential for helping businesses train data to accelerate their machine learning applications. He shares his insights into the state of the AI with our partner Mike Volpi.
Mike volpi — You’ve been studying machine learning since high school, and you’ve interacted with many clients and experts, including those you’ve recruited at Scale. Give us your state of the union for AI. Tell us about the maturity of the technology, its adoption, and the sector right now.
Alex wang — Deep learning and neural networks are fairly mature. They’re ready to be applied to practical problems and work incredibly well on the types of data that we didn't have a handle on before, such as images, text, audio, and video. Deep learning around convolutional neural nets and recurrent neural networks is also very much ready for production at this point. Autonomous vehicles rest upon a lot of this deep, supervised learning, as do Amazon Go-style systems, Siri, and other voice systems, though we're at the tip of the iceberg in terms of applications. And then there's the cutting edge research around reinforcement learning with systems that can play games really well, or what people would call sort of the path to AGI.
What we’re really focused on at Scale is taking current deep learning technology and deploying it very broadly throughout the world, and that’s a really tough challenge. There are ethical questions, like what does it really mean to have these systems control much of our lives, and operational questions too, like how can we ensure oversight of deep learning systems, and deal with issues like bias. As a technologist, I think of these as implementational details, but I’m still super excited about the potential of applying this technology to the long tail of real-world problems. We talk a lot about the impact of mobile and of the internet, even of the computer itself, but AI will be huge, and we’re very, very early in this new wave of technology.
Mike — What kind of timeframe would you put on AI? Are we in year one, and is it a 10-year transformation, or a 20-year one?
Alex — The rate of progress in research is really incredible, and we’ll see a lot more breakthroughs in the next ten years, but I think the broader arc of transformation will be more like 20 years. There are few at-scale applications today: voice assistants are a massive one, and facial recognition technology too —both are really large-scale things already being used around the world. We don’t yet have the ‘Uber’ of AI, but it will happen.
Mike — What would you say right now are the biggest challenges to the deployment and operationalization of AI?
Alex — Figuring out the great use cases. And that’s hard because it means understanding what the limits of the technology are. Even the term ‘artificial intelligence’ implies a much greater capability than the actual technology has today. So the people designing the applications have to be pretty judicious about understanding what use cases are appropriate. Once they have the use case, then they have to build the model and the machine learning around it. I think that the process is still very hard for most people and organizations. Then we have to address the issues that the big tech companies are dealing with — like ethics, bias, and explainability.
Added to that is the challenge of media coverage, which often takes advancements and extrapolates those to infinity. In reality, the technology is much more limited. So yes — we need more people to understand the limits of the technology and how to apply it judiciously.
Mike — A common AI thread is this issue of people's jobs disappearing, like taxi drivers, truck drivers and the eight million people in America who drive some form of a commercial vehicle. What's your view on the implications that AI has for jobs?
— What would you say right now are the biggest challenges to the deployment and operationalization of AI?
Alex — It's a simple mental model: there are all these people who do this work today and then a switch suddenly flips and all that work will be automated. That’s how some of the media paints it. Yet change never happens instantly, and the reality is that the technology we do build will be much more limited, and will initially make these jobs easier.
Think of the ATM. People thought that once ATMs arrived, bank tellers would be out of a job. But that's not what happened. The number of bank tellers in the US actually went up considerably. ATMs allowed them to focus on the higher value parts of their job, which meant the company saw them as more valuable. ATMs also enabled growth in the banking sector, so the number of bank tellers had to grow. The impact of automation or machine learning is complex and nuanced, but actually more likely to make jobs easier while allowing people to focus on higher-value aspects of their work.
Mike — Should we be worried about Elon's Robocalypse? Are we going to be working for the machines, or are the machines working for us?
Alex — Well, any machine can just be turned off! I’d also point out that a lot of people are working on automating services that will help people. Medicine is a great example — there’s a 10x shortage of doctors globally, and a lot of people aren’t able to access good medical care. So machine learning in healthcare doesn’t necessarily mean automating medicine in the US but could mean providing healthcare to people around the world.
Mike — In business, the big tech companies are already benefiting from their use of AI, but what's your view on new businesses that are going to be created around AI?
Alex — AI is different from a lot of the technological revolutions we've had in the past because it’s a more capital-intensive technology to build a business around than the internet was, or indeed mobile. It demands that you hire smart and expensive people, but you also need data, and some serious computing infrastructure. It's more expensive to build an AI business than most other kinds of tech businesses.
There's going to be sort of two kinds of AI businesses: ones that try to solve a problem vertically for an end customer who might understand nothing about AI, and then platforms who build technologies that help other companies deploy this technology to their end customers.
At Scale, we believe there’s a huge opportunity around this platform play. There's so much we can do to make AI easier for other organizations to deploy. We look at how they’re managing all their data, how they are trading and testing models, and we want to make it easier for businesses who understand where AI can really yield an impact.
Mike — Even an existing company, a hotel business, an airline or a package delivery business— they all have data that is proprietary to them as well.
Alex — Exactly. There’s a massive opportunity for a lot of pre-existing businesses that have huge amounts of interesting data already collected. They just need to build technology around it.
Mike — You often talk about how data is the new gold in terms of value creation in AI. But if Google, Amazon, and Facebook have all the data, what does that mean for smaller companies? Are they always going to be second best?
Alex — The reality is that there are always going to be niches, and there are always things that are outside the purview of these big companies— things that are really important but that they're just not focused on. Large technology companies have a lot of data about their consumers and how those people use their products, yet usually very little data outside of that. So if you wanted to start a medical imagery company, you're likely to start on a level playing field with a big tech company.
The strategy for AI companies thinking about data strategy is to pursue something niche, where there’s a strong need, and where the big tech companies aren’t looking. Then you have an opportunity to build your own data advantage. Ultimately there'll be many, many billion-dollar companies created with AI as their core technology.
Published — Feb. 26, 2020